The Importance of Encoding Versus Training with Sparse Coding and Vector Quantization
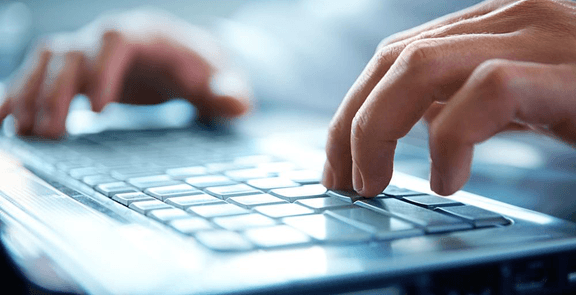
While vector quantization (VQ) has been applied widely to generate features for visual recognition problems, much recent work has focused on more powerful methods. In particular, sparse coding has emerged as a strong alternative to traditional VQ approaches and has been shown to achieve consistently higher performance on benchmark datasets. Both approaches can be split into a training phase, where the system learns a dictionary of basis functions, and an encoding phase, where the dictionary is used to extract features from new inputs. In this work, we investigate the reasons for the success of sparse coding over VQ by decoupling these phases, allowing us to separate out the contributions of training and encoding in a controlled way. Through extensive experiments on CIFAR, NORB and Caltech 101 datasets, we compare several training and encoding schemes, including sparse coding and a form of VQ with a soft threshold activation function. Our results show not only that we can use fast VQ algorithms for training, but that we can just as well use randomly chosen exemplars from the training set. Rather than spend resources on training, we find it is more important to choose a good encoder—which can often be a simple feed forward non-linearity. Our results include state-of-the-art performance on both CIFAR and NORB.