Semantic Compositionality through Recursive Matrix-Vector Spaces
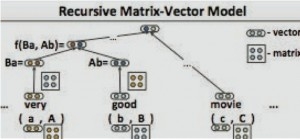
Single-word vector space models have been very successful at learning lexical information. However, they cannot capture the compositional meaning of longer phrases, preventing them from a deeper understanding of language. We introduce a recursive neural network (RNN) model that learns compositional vector representations for phrases and sentences of arbitrary syntactic type and length. Our model assigns a vector and a matrix to every node in a parse tree: the vector captures the inherent meaning of the constituent, while the matrix captures how it changes the meaning of neighboring words or phrases. This matrix-vector RNN can learn the meaning of operators in propositional logic and natural language. The model obtains state of the art performance on three different experiments: predicting fine-grained sentiment distributions of adverb-adjective pairs; classifying sentiment labels of movie reviews and classifying semantic relationships such as cause-effect or topic-message between nouns using the syntactic path between them.