Learning Word Vectors for Sentiment Analysis
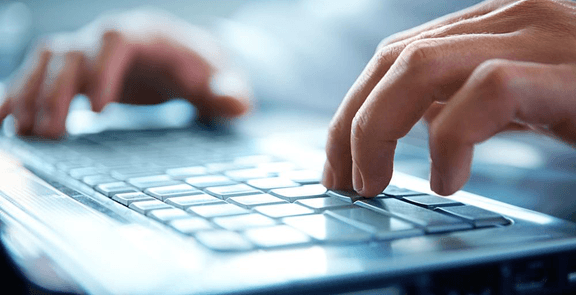
Unsupervised vector-based approaches to semantics can model rich lexical meanings, but they largely fail to capture sentiment information that is central to many word meanings and important for a wide range of NLP tasks. We present a model that uses a mix of unsupervised and supervised techniques to learn word vectors capturing semantic term–document information as well as rich sentiment content. The proposed model can leverage both continuous and multi-dimensional sentiment information as well as non-sentiment annotations. We instantiate the model to utilize the document-level sentiment polarity annotations present in many online documents (e.g. star ratings). We evaluate the model using small, widely used sentiment and subjectivity corpora and find it out-performs several previously introduced methods for sentiment classification. We also introduce a large dataset of movie reviews to serve as a more robust benchmark for work in this area.