Convergence rates of the Voting Gibbs classifier, with application to Bayesian feature selection
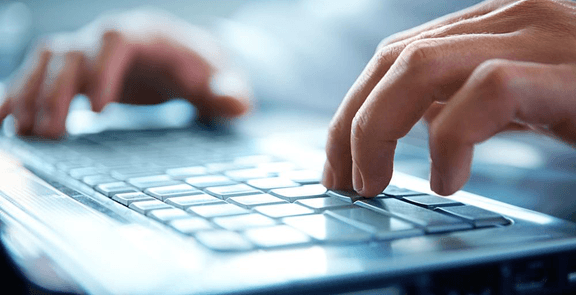
The Gibbs classifier is a simple approximation to the Bayesian optimal classifier in which one samples from the posterior for the parameter », and then classifies using the single classifier indexed by that parameter vector. In this paper, we study the Voting Gibbs classifier, which is the extension of this scheme to the full Monte Carlo setting, in which N samples are drawn from the posterior and new inputs are classified by voting the N resulting classifiers. We show that the error of Voting Gibbs converges rapidly to the Bayes optimal rate; in particular the relative error decays at a rapid O(1/N) rate. We also discuss the feature selection problem in the Voting Gibbs context. We show that there is a choice of prior for Voting Gibbs such that the algorithm has high tolerance to the presence of irrelevant features. In particular, the algorithm has sample complexity that is logarithmic in the number of irrelevant features.