A Probabilistic Model for Semantic Word Vectors
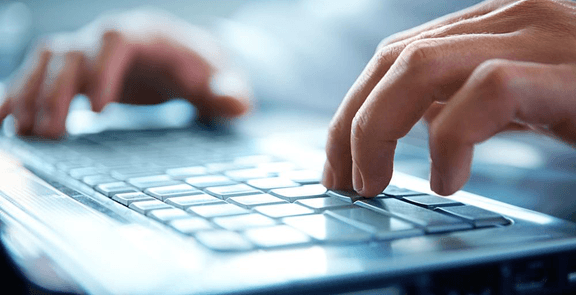
Vector representations of words capture relationships in words’ functions and meanings. Many existing techniques for inducing such representations from data use a pipeline of hand-coded processing techniques. Neural language models offer principled techniques to learn word vectors using a probabilistic modeling approach. However, learning word vectors via language modeling produces representations with a syntactic focus, where word similarity is based upon how words are used in sentences. In this work we wish to learn word representations to encode word meaning – semantics. We introduce a model which learns semantically focused word vectors using a probabilistic model of documents. We evaluate the model’s word vectors in two tasks of sentiment analysis.