A Probabilistic Approach to Mixed Open-loop and Closed-loop Control, with Application to Extreme Autonomous Driving
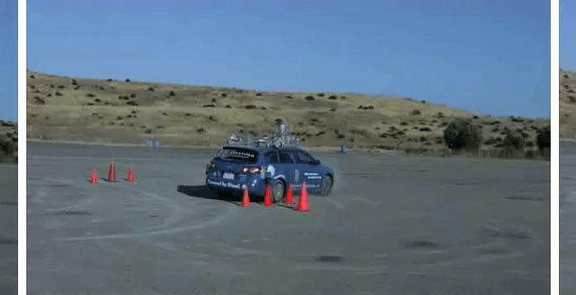
We consider the task of accurately controlling a complex system, such as autonomously sliding a car sideways into a parking spot. Although certain regions of this domain are extremely hard to model (i.e., the dynamics of the car while skidding), we observe that in practice such systems are often remarkably deterministic over short periods of time, even in difficult-to-model regions. Motivated by this intuition, we develop a probabilistic method for combining closed-loop control in the well-modeled regions and open-loop control in the difficult-to-model regions. In particular, we show that by combining 1) an inaccurate model of the system and 2) a demonstration of the desired behavior, our approach can accurately and robustly control highly challenging systems, without the need to explicitly model the dynamics in the most complex regions and without the need to hand-tune the switching control law. We apply our approach to the task of autonomous sideways sliding into a parking spot, and show that we can repeatedly and accurately control the system, placing the car within about 2 feet of the desired location; to the best of our knowledge, this represents the state of the art in terms of accurately controlling a vehicle in such a maneuver.