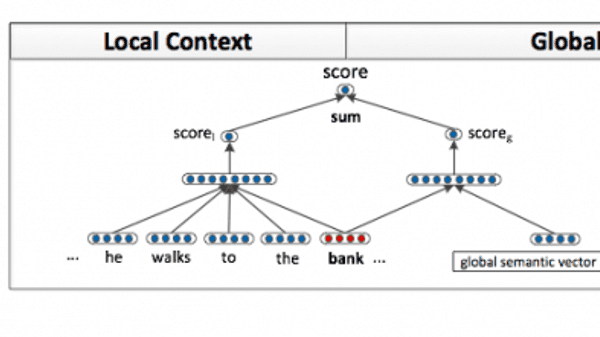
Improving Word Representations via Global Context and Multiple Word Prototypes
We present a new neural network architecture which 1) learns word embeddings that better capture the semantics of words by incorporating both local and global document context, and 2) accounts for homonymy and polysemy by learning multiple embeddings per word. Eric H. Huang, Richard Socher, Christopher D. Manning and Andrew Y. Ng in ACL 2012.